How Does AI Detection Work?
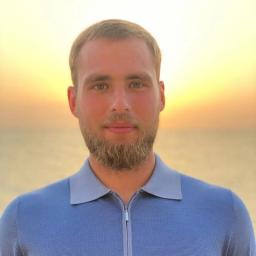
Dan is an entrepreneur with a rich tech background and a passion for AI, known for his global perspective and leadership.
How Does AI Detection Work?
AI detection refers to the process of distinguishing between human-created content and content generated by AI systems, such as language models, chatbots, or image-generation algorithms.AI detection has become a critical tool in various industries, serving a wide range of purposes. Some of the key areas where AI detection plays a crucial role include:Academic Integrity
Educational institutions rely on AI detectors to identify and prevent the use of AI-generated essays, research papers, and other forms of academic dishonesty.Journalistic Integrity
News organizations and media outlets use AI detection to ensure the authenticity of news articles, preventing the spread of AI-generated fake news and misinformation.Intellectual Property Protection
Companies and individuals employ AI detection techniques to safeguard their intellectual property, such as copyrighted text, images, or code, from unauthorized AI-based reproduction or manipulation.Social Media Moderation
Social media platforms leverage AI detection algorithms to identify and remove AI-generated spam, bots, and malicious content, maintaining a safer and more trustworthy online environment.Cybersecurity
AI detection plays a vital role in identifying and preventing AI-powered cyberattacks, such as deepfake phishing attempts or AI-assisted malware, enhancing overall cybersecurity measures.What Are AI Detectors Used For in the Legal Space?
From identifying AI-generated documents to detecting deepfakes and manipulated evidence, AI detectors are becoming increasingly important tools for legal professionals. Some of the key applications of AI detectors in the legal space are:Evidence Authentication
AI detectors are used to verify the authenticity of digital evidence submitted in court. They can identify AI-generated documents, images, or videos that may have been tampered with or fabricated, ensuring that only genuine evidence is admissible in legal proceedings.Fraud Detection in Legal Documents
AI detectors can identify fraudulent legal documents, such as contracts, wills, or affidavits, that may have been generated or manipulated using AI tools. By detecting anomalies and patterns associated with AI-generated content, legal professionals can prevent the use of fraudulent documents and protect the integrity of legal transactions.Intellectual Property Protection
In intellectual property disputes, AI detectors can help identify AI-generated content that may infringe upon copyrights, trademarks, or patents. They can detect AI-generated text, images, or videos that closely resemble or copy protected works, assisting in the enforcement of intellectual property rights.Deepfake Detection
AI detectors play a vital role in identifying deepfakes, which are AI-generated videos or audio recordings that convincingly impersonate real individuals. In legal contexts, deepfakes can be used to manipulate evidence or create false testimonies. AI detectors help expose deepfakes and prevent their use in legal proceedings.Specific Use Cases of AI Detection in the Legal Field
Legal organizations and regulatory bodies may need to develop guidelines and best practices for the use of AI detectors in legal proceedings. This includes establishing standards for the admissibility of AI-generated evidence, defining the roles and responsibilities of legal professionals in using AI detectors, and ensuring the transparency and explainability of AI detection methods.Contract Review and Analysis
AI detectors can assist legal professionals in reviewing and analyzing contracts by identifying AI-generated clauses or terms that may be fraudulent, misleading, or inconsistent with legal standards. This helps ensure the integrity and enforceability of legal agreements.E-Discovery and Document Review
In the process of e-discovery, AI detectors can help identify AI-generated documents that may be relevant to a legal case. By distinguishing between human-created and AI-generated content, legal teams can more efficiently review and analyze large volumes of electronic evidence.Forensic Analysis and Investigation
AI detectors are used in forensic investigations to identify AI-generated evidence, such as manipulated images or videos, that may be used to mislead or deceive investigators. By detecting AI-generated content, forensic experts can ensure the accuracy and reliability of their findings.Litigation Strategy and Risk Assessment
AI detectors can assist legal teams in assessing the risks and potential challenges associated with AI-generated evidence in litigation. By understanding the capabilities and limitations of AI detectors, lawyers can develop effective strategies for presenting or challenging evidence in court.Implications for Legal Ethics and Regulations
The use of AI detectors in the legal industry raises important ethical and regulatory considerations. As AI technologies advance, legal professionals must stay informed about the capabilities and limitations of AI detectors and ensure their use complies with legal and ethical standards.How Reliable Are AI Detectors?
The reliability of AI detectors depends on several key aspects, including data quality, model complexity, and the presence of adversarial attacks.Data Quality and Representativeness
The performance of AI detectors heavily relies on the quality and representativeness of the training data. If the training data is biased, noisy, or lacks diversity, the detector may struggle to generalize well to new, unseen instances of AI-generated content.Model Complexity and Interpretability
Complex AI detection models, such as deep neural networks, can capture intricate patterns in data but may suffer from a lack of interpretability. Simpler models, such as decision trees or rule-based systems, are more interpretable but may not be as effective in capturing subtle nuances in AI-generated content.Adversarial Attacks and Evasion Techniques
AI detectors are vulnerable to adversarial attacks, where malicious actors intentionally manipulate AI-generated content to evade detection. Adversarial examples can be crafted by introducing subtle perturbations or by exploiting weaknesses in the detection models.To assess the reliability of AI detectors, various performance metrics are used:Accuracy
Accuracy measures the overall correctness of the detector's predictions, indicating the percentage of instances that are correctly classified as either human-created or AI-generated.Precision and Recall
Precision quantifies the proportion of true positive predictions among all positive predictions, while recall measures the detector's ability to identify all instances of AI-generated content correctly. A high precision indicates a low false-positive rate, while a high recall suggests a low false-negative rate.Cross-Validation and Testing on Unseen Data
To ensure the detector's reliability, it is essential to evaluate its performance using cross-validation techniques and by testing it on unseen data. This helps assess the detector's ability to generalize and perform well on new, previously unseen instances of AI-generated content.How To Improve AI Detector Reliability
Several strategies can be employed to enhance the reliability of AI detectors:Ensemble Methods and Model Combination
Ensemble methods combine multiple detection models to make more robust and accurate predictions. By aggregating the outputs of different models, ensemble methods can reduce the impact of individual model weaknesses and improve overall reliability.Continuous Monitoring and Updating
AI detectors need to be continuously monitored and updated to adapt to the ever-evolving landscape of AI-generated content. Regular retraining and fine-tuning of detection models using the latest data can help maintain their effectiveness over time.Adversarial Training and Robustness
To mitigate the impact of adversarial attacks, AI detectors can be trained using adversarial examples. By exposing the models to deliberately crafted evasive content during training, they can learn to be more robust against such attacks.Explainable AI Techniques
Incorporating explainable AI techniques into detection models can provide insights into the decision-making process and help identify potential weaknesses or biases. Explainable AI methods, such as feature importance analysis or rule extraction, can enhance the interpretability and trustworthiness of AI detectors.AI Detectors vs. Plagiarism Checkers
While AI detectors aim to identify content generated by artificial intelligence, plagiarism checkers focus on detecting instances of copied or plagiarized content. Understanding the similarities and differences between these two approaches is crucial for effectively using them in various contexts.Similarities
Text Analysis: Both AI detectors and plagiarism checkers rely on analyzing textual content to identify patterns, similarities, or anomalies.Feature Extraction: Both tools extract relevant features from the text, such as word frequencies, n-grams, or stylometric attributes, to represent the content in a machine-readable format.Machine Learning: Some advanced plagiarism checkers may incorporate machine learning algorithms to improve their detection capabilities, similar to AI detectors.Differences
Reference Database:: Plagiarism checkers typically compare the input text against a vast database of existing content to find matches and identify potential instances of plagiarism. AI detectors, on the other hand, focus on identifying patterns and characteristics specific to AI-generated content, without necessarily relying on a reference database.Pattern Recognition: AI detectors are designed to recognize patterns and features that are unique to AI-generated content, such as certain linguistic structures, word choices, or stylistic elements. Plagiarism checkers, in contrast, look for exact or near-exact matches between the input text and the content in their reference database.Scope: Plagiarism checkers are primarily concerned with detecting copied or plagiarized content, while AI detectors have a broader scope, aiming to identify any content generated by AI, regardless of whether it is plagiarized or not.While AI detectors and plagiarism checkers serve different primary purposes, combining both approaches can lead to more comprehensive and effective content screening. By using AI detectors to identify AI-generated content and plagiarism checkers to detect copied or plagiarized material, organizations can ensure a higher level of content integrity and authenticity.Challenges With AI Detection
AI detector challenges include adversarial attacks, concept drift, and the trade-off between false positives and false negatives. Understanding and addressing these challenges is crucial for the development and deployment of robust AI detection systems.Adversarial Attacks and Evasion Techniques
Adversarial attacks pose a major challenge to AI detectors. These attacks involve malicious actors intentionally manipulating AI-generated content to evade detection. By introducing subtle perturbations or crafting content that exploits weaknesses in the detection models, adversaries can create AI-generated content that appears legitimate and bypasses the AI detectors.Obfuscation and Encryption
Adversaries may use obfuscation techniques to hide the telltale signs of AI-generated content. This can involve encrypting the content, using steganography to embed hidden messages, or employing other methods to make the content appear more human-like and difficult to detect.Mimicry and Impersonation
Adversarial attacks can also involve mimicking the style, language, or patterns of human-created content to evade detection. By carefully studying and replicating the characteristics of genuine content, adversaries can create AI-generated content that closely resembles human-created content, making it harder for AI detectors to distinguish between the two.Concept Drift and Evolving AI Systems
Concept drift refers to the phenomenon where the characteristics and patterns of AI-generated content change over time as AI technologies evolve and improve. This poses a significant challenge for AI detectors, as the models trained on older datasets may become less effective or hallucinate in detecting newer, more sophisticated AI-generated content.Balancing False Positives and False Negatives
AI detectors often face a trade-off between false positives (incorrectly flagging human-created content as AI-generated) and false negatives (failing to detect actual AI-generated content). Striking the right balance between these two types of errors is a significant challenge.On one hand, a high rate of false positives can lead to genuine content being wrongly flagged or removed, causing frustration and inconvenience for users or content creators. On the other hand, a high rate of false negatives allows AI-generated content to slip through undetected, undermining the integrity and trustworthiness of the content.In some cases, the cost of false negatives may be higher, warranting a more conservative approach to detection. In other cases, such as in social media moderation, a higher tolerance for false positives may be acceptable to ensure a safer online environment.Legal and Ethical Considerations
AI detectors often rely on analyzing large amounts of data, including personal information, to train and improve their models. This raises significant privacy concerns, as individuals may not be aware of how their data is being collected, used, or shared for AI detection purposes.To address these concerns, organizations must comply with relevant data protection regulations, such as the General Data Protection Regulation (GDPR) in the European Union and the California Consumer Privacy Act (CCPA) in the United States. These regulations set forth requirements for obtaining consent, ensuring data security, and providing individuals with the right to access, correct, or delete their personal data.When developing and deploying AI detectors, organizations must implement robust data protection measures, such as data minimization, encryption, ethics, and secure storage, to safeguard individuals' privacy rights. They should also provide clear and transparent information about their data practices, allowing individuals to make informed decisions about the use of their data for AI detection purposes.Bias and Fairness in AI Detection Systems
AI detectors, like any other AI system, can be subject to biases that may lead to unfair or discriminatory outcomes. These biases can arise from various sources, such as biased training data, flawed model design, or the inherent biases of the developers and users of the systems.Algorithmic bias in AI detectors can result in certain groups of individuals being disproportionately flagged or misclassified as AI-generated content creators, leading to unfair treatment or discrimination.To mitigate bias and ensure fairness in AI detection systems, organizations must take proactive steps, such as:Diverse and Representative Training Data
Ensuring that the training data used to develop AI detectors is diverse, representative, and free from biases. This includes collecting data from a wide range of sources and demographics to capture the full spectrum of human-created content.Fairness Metrics and Auditing
Incorporating fairness metrics and regularly auditing AI detection systems to identify and address any biases or disparities in performance across different groups. This involves analyzing the detection rates, false positives, and false negatives for various demographics and taking corrective actions when necessary.Inclusive Design and Development
Fostering diversity and inclusivity in the teams responsible for designing, developing, and deploying AI detectors. By bringing together individuals with diverse backgrounds, perspectives, and experiences, organizations can help identify and mitigate potential biases in the development process.Transparency and Explainability of AI Detection Models
Transparency and explainability are critical considerations in the development and use of AI detection models. As these models become more complex and sophisticated, it becomes increasingly challenging to understand how they arrive at their decisions and what factors influence their outputs.The lack of transparency and explainability in AI detectors can lead to a lack of trust and accountability. If individuals or organizations are subject to decisions made by AI detectors, they have a right to know how those decisions were reached and to challenge them if necessary.To address these concerns, there is a growing emphasis on developing explainable AI (XAI) techniques that can provide insights into the decision-making process of AI detectors. XAI methods aim to make AI models more interpretable and understandable by providing explanations for their predictions or highlighting the key factors that influenced their outputs.Want to Learn More?
The ability to distinguish between human-created and AI-generated content is crucial for maintaining the integrity, trustworthiness, and authenticity of information in various domains, from academia and journalism to legal proceedings and beyond.For more information on how AI is changing the legal field, visit our AI page for more in-depth articles.Tired of spending hours working on document review, legal contract summarization, due diligence, and other routine tasks?
Discover how lawyers like you are using our AI platform.