Bias Detection In AI
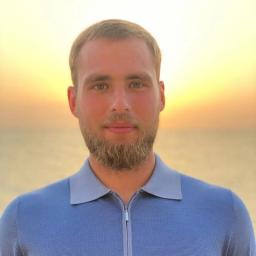
Dan is an entrepreneur with a rich tech background and a passion for AI, known for his global perspective and leadership.
Understanding AI bias, its origins, types, and where it occurs, is important for legal professionals who increasingly rely on AI tools. Equally important is exploring strategies to mitigate these biases, ensuring that AI aids rather than impedes the pursuit of justice.We aim to arm legal practitioners with the knowledge to navigate, scrutinize, and leverage AI technologies effectively, fostering an environment where technological advancements and ethical considerations are inextricably linked. By addressing AI bias head-on, the legal field can utilize the full potential of AI to serve justice fairly and equitably, ensuring that technology acts as a force for good, bridging gaps rather than widening them.What Is AI Bias?
AI bias refers to systematic and non-random errors in decision-making processes facilitated by AI systems that lead to unfair outcomes, such as privileging one arbitrary group of users over others.AI systems learn from vast datasets, extracting patterns and making predictions based on the information provided during their training phase. Ideally, these predictions should be objective and unbiased.If the input data contain biases—whether through skewed representation, historical inequalities, or subjective decision-making—these biases will be embedded within the AI's decision-making processes. Thus, instead of acting as neutral arbiters, AI systems can inadvertently become conduits for perpetuating historical injustices.Understanding AI bias requires acknowledging that technology is not developed in a vacuum. AI systems are created by humans, and as such, they can inherit human prejudices.Recognizing AI bias is the first step towards addressing and mitigating its effects, ensuring that AI technologies serve justice and fairness, rather than undermining them. In the next sections, we'll explore the root causes of AI bias and delve deeper into how these biases can affect the legal field, offering insights into prevention and mitigation strategies.What Causes AI Bias?
AI bias stems from a variety of sources, each contributing to the potential for skewed outcomes in AI-driven decision-making. Understanding these sources is crucial for legal professionals and technologists alike, as it informs the strategies needed to mitigate bias. Here are the primary causes of AI bias:1. Biased Data Sources
AI systems learn to make decisions based on the data they are fed. If this data is not representative of the population or contains historical biases, the AI will likely reproduce or even amplify these biases.For instance, data on past convictions might reflect societal biases against certain racial or economic groups. When AI systems are trained on such data to predict future criminal behavior or set bail amounts, they may unfairly target these groups.2. Human Prejudices in Data Labeling
The process of labeling data, which involves categorizing or annotating data to train AI models, is often carried out by humans. This step can introduce subjective biases into the system. For example, if individuals labeling data hold conscious or unconscious biases about what constitutes a "risky" individual in the context of bail decisions, this bias will be transmitted to the AI system.3. Algorithmic Design Choices
The algorithms themselves, designed by humans, can incorporate biases through the assumptions made during their development. Choices regarding which variables to include, which data points to prioritize, and how to analyze different elements can all influence the outcomes in biased ways. The decision to prioritize certain indicators of recidivism over others in an AI tool can result in biased assessments that affect people's lives and liberties.4. Lack of Diversity Among AI Developers
The field of AI development has been critiqued for its lack of diversity. When development teams lack diverse perspectives, the systems they create are more likely to overlook or inadequately address the needs and realities of underrepresented groups. This lack of diversity can inadvertently lead to the development of systems that do not fully account for the variety of human experiences and conditions, thereby entrenching existing inequalities.5. Feedback Loops
AI systems can also be caught in feedback loops, where biased predictions lead to actions that reinforce those biases. For example, if an AI system in the legal field predicts higher crime rates in a particular area and law enforcement resources are consequently concentrated there, the increased likelihood of arrests in that area can be fed back into the system, falsely confirming the initial bias.Where Can AI Bias Occur?
AI bias can occur at multiple stages of the AI system development and deployment lifecycle. This section breaks down the key stages: Data Collection, Data Labeling, Model Training, and Deployment, providing insights into how biases at each stage can impact the fairness and integrity of AI systems.Data Collection
If the datasets are not representative of all groups or fail to capture the diversity of real-world scenarios, the resulting AI system can exhibit biases.Data reflecting past decisions, actions, or outcomes often contain historical biases. AI systems trained on data like this may perpetuate or amplify these biases unless corrective measures are taken. This could affect bail recommendation systems, risk assessment tools, and more, embedding historical injustices into future decisions.Data Labeling
The process of labeling involves assigning values or categories to data points, which is often done manually by humans. Subjective biases can influence how data is labeled, affecting the AI's learning process. This might impact how cases are categorized for predictive analysis, potentially introducing biases into predictions about case outcomes or recidivism risks.Variability in labeling standards and practices can introduce inconsistencies, leading to biased or unreliable AI outputs. One must ensure uniformity and clarity in how data is labeled in order to reduce AI bias.Model Training
The choice of algorithms and the criteria for their optimization can embed biases into AI systems. Algorithms that prioritize certain outcomes or patterns over others can lead to biased decision-making processes or AI hallucinations, affecting everything from legal research tools to predictive policing systems.AI systems may overfit to the biases present in their training data, resulting in models that perform well on similar biased data but poorly in unbiased, real-world scenarios. This is particularly problematic in scenarios where fairness and accuracy are paramount.Deployment
The deployment of AI systems in environments or for purposes different from those they were originally designed for can reveal biases not apparent during testing. Legal professionals must ensure that AI tools are used within the appropriate context and continuously monitor their performance to identify and correct biases.Feedback Loops
AI systems in operation can create feedback loops that reinforce initial biases. For instance, an AI system used for allocating law enforcement resources might direct more patrols to areas it predicts as high crime, thereby increasing arrests in those areas and reinforcing the system's original bias.Types of AI Bias
This section categorizes common biases into Training Data Bias, Algorithmic Bias, Confirmation Bias, Decision-Making Bias, and Social Bias, illustrating how each type can affect the fairness and integrity of legal AI applications.Training Data Bias
Training data bias occurs when the data used to train an AI model are not representative of the broader population or situation to which the AI system will be applied. This type of bias can lead to AI systems that perform well for certain groups but inadequately for others.If predictive policing tools are trained on arrest records from neighborhoods with historically high police presence, they may unfairly target these communities, perpetuating cycles of over-policing.Algorithmic Bias
Algorithmic bias refers to biases that are introduced by the way an AI algorithm is designed or the choices made during its development. This can include the selection of variables, the weighting of different factors, or the optimization goals set for the model.For instance, an AI system designed to predict the outcomes of parole hearings might prioritize factors that inadvertently disadvantage certain demographic groups, such as employment history, without considering systemic barriers to employment.Confirmation Bias
Confirmation bias in AI occurs when a model disproportionately favors information that confirms the pre-existing beliefs or biases encoded in its training data or algorithm. This can lead to a self-reinforcing cycle where the AI system continues to make decisions that reflect and strengthen these biases.In legal research, for example, an AI tool that suggests resources based on previous queries might continually direct users towards opinions or cases that align with a certain viewpoint, overlooking a broader range of relevant legal precedents.Decision-Making Bias
Decision-making bias in AI systems refers to biases that affect the judgments or predictions made by these systems. This type of bias can result from a combination of training data bias, algorithmic bias, and confirmation bias, leading to unfair or discriminatory outcomes.Decision-making bias might manifest in recommendations for harsher sentences for individuals based on biased data or flawed algorithmic assessments of risk.Social Bias
Social bias is the reflection of societal stereotypes and inequalities in AI systems. This bias often mirrors existing prejudices related to race, gender, ethnicity, socioeconomic status, or other social categories.Social bias in legal AI applications can exacerbate inequalities, such as through biased legal document analysis tools that fail to equally recognize the relevance or importance of cases involving underrepresented groups.Transparency
Transparency in AI involves understanding how AI systems make decisions, what data they use, and the rationale behind their outputs. Legal professionals should:Demand clarity from AI vendors about how their tools work, including the data sources, algorithms, and decision-making processes used.Advocate for explainable AI that provides understandable explanations for its recommendations, predictions, and decisions, enabling lawyers to critically assess the reliability and fairness of AI outputs.Efficiency
To ensure efficiency enhancements are balanced with ethical considerations, lawyers should:Prioritize bias assessment as part of the efficiency evaluation, ensuring that faster outcomes do not perpetuate unfair practices.Use AI as a tool to augment, not replace, human decision-making, maintaining a critical oversight role to catch and correct potential biases.Fairness
Achieving fairness in AI requires active efforts to identify and mitigate biases at every stage of AI development and deployment. Lawyers can contribute by:Implementing bias audits for AI systems before adoption and regularly thereafter, using a diverse set of metrics to assess fairness across different groups.Collaborating with interdisciplinary teams including ethicists, sociologists, and technologists, to develop more equitable AI solutions.Human Supervision
Human supervision is a key component in mitigating bias in AI. Lawyers should ensure:Decisions influenced by AI undergo human review, particularly in high-stakes situations like sentencing, bail decisions, or case outcomes.Continuous training for legal professionals on the potential biases of AI tools and how to effectively use them and supervise these technologies and consider their recommendations critically.Actionable Steps To Mitigate Bias
Participate in or organize training sessions on ethics in AI and bias mitigation strategies.Engage with AI developers and vendors to express the need for bias-reduction features and transparency in AI legal tools.Advocate for regulations and standards that enforce fairness, accountability, and transparency in AI applications within the legal system.Examples Of Bias In AI
To contextualize the discussion on AI bias and its mitigation, here are some real-world examples where AI bias has manifested, particularly within the legal and related fields.Predictive Policing Systems
Predictive policing tools have been criticized for reinforcing racial biases. In some cases, these systems have disproportionately targeted minority communities by predicting higher crime rates in areas with historical over-policing.This creates a feedback loop where increased police presence leads to more arrests, which in turn are fed back into the system, falsely validating its original predictions. Such bias not only undermines the fairness of law enforcement efforts but also erodes public trust in the justice system.Sentencing Algorithms
In the United States, algorithms used to assess the risk of recidivism have come under scrutiny for potential biases against racial minorities. Investigations have found that some of these tools assign higher risk scores to black defendants than to white defendants, even when controlling for criminal history and future criminal behavior. This can lead to harsher sentencing recommendations for minorities, perpetuating racial disparities within the justice system.Hiring Tools
Several companies have reported that their AI systems developed biases against women or certain ethnic groups because they were trained on historical hiring data that reflected these biases.Similar biases could affect hiring practices within law firms or legal departments, potentially discriminating against qualified candidates based on gender, ethnicity, or other irrelevant factors.Credit Scoring Algorithms
Credit scoring algorithms, which influence decisions on loan approvals, credit limits, and interest rates, have shown biases against minority and low-income applicants. These biases stem from historical data and socioeconomic factors that disproportionately affect these groups.Similar biases could influence decisions on client eligibility for legal aid or representation, impacting access to legal services.The Future of AI Bias
Through concerted efforts to advance technological solutions, strengthen regulatory frameworks, educate stakeholders, and foster collaboration, we can aim to minimize bias in AI.The goal is a future where AI systems augment human capabilities and do so in a way that reflects the best of our values—fairness, equality, and respect for all individuals. By addressing AI bias head-on, we ensure that the legacy of AI is one of positive transformation, enriching society while safeguarding the rights and dignity of every person.Learn More
Check out the rest of our articles to discover how AI and trying DocuEase can transform your legal practice and prepare you for the next wave of legal technology advancements.Tired of spending hours working on document review, legal contract summarization, due diligence, and other routine tasks?
Discover how lawyers like you are using our AI platform.